Data governance and ethical use of AI
To have a working AI algorithm, you need two things: historical data and the definition of success. You train the algorithm so that it understands what is associated with success. Although we tend to believe that algorithms are scientific and objective, the truth is a bit different.
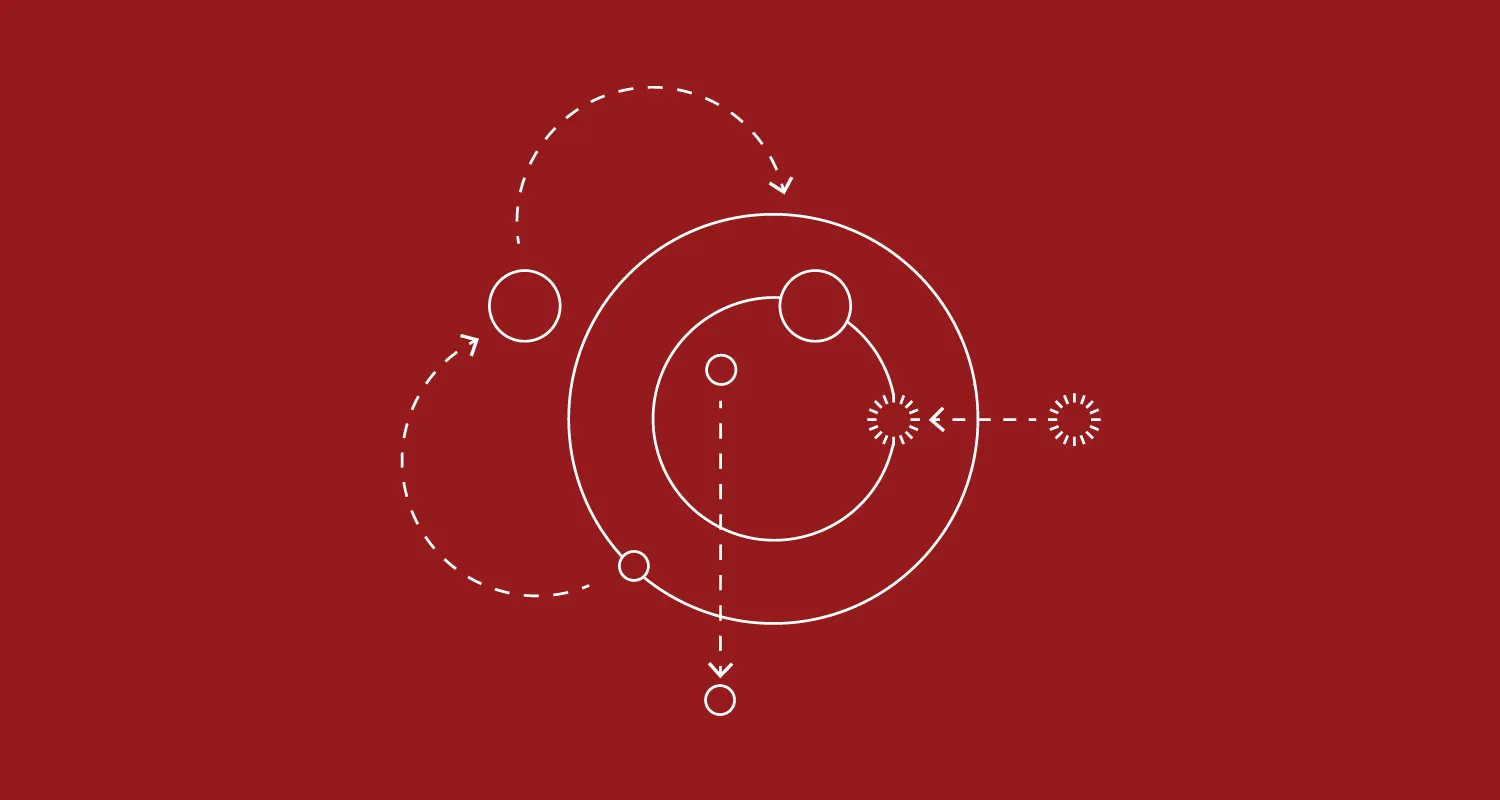
Cathy O'Neil, author of “Weapons of Math Destruction,” works on raising awareness about the usually mysterious and often unjust ways algorithms work. This is especially important in cases where people’s lives are impacted.
Take the COMPAS recidivism algorithm used in the criminal justice system, for example. Because it’s based on biased data, it scores African American criminals with a higher number, indicating a high probability that they will repeat the felony. This impacts the fairness of their trials. As a matter of fact, statistics have shown that they get longer sentences when the algorithm scores them higher.
So, how do you prevent AI's destructive force? How can you ensure that you’re building and using AI models ethically?
Privacy and AI
AI systems require vast amounts of data to work properly. Like we said earlier, you feed them with historical data and define what success means. So, what kind of data are we talking about?
The average user doesn’t really think too much about their digital footprint or the privacy of their data. For example, did you know that ChatGPT collects account-level information? This includes your email address, IP address, device, location, and more. The model itself has been trained from a diverse range of sources across the internet – articles, books, and social media posts included.
Organizations need to be transparent about how they collect, use, and store data. This includes public information on the data collected, its purpose, and who has access to it. Users need to give their explicit consent to organizations, and they should also have control over their data.
Regulations like the General Data Protection Regulation (GDPR) and the California Consumer Privacy Act (CCPA) exist to protect users’ data and ensure ethical handling. This is true for all data collection practices, not just AI model training. Users have the lawful right to opt out of the sale of their data.
So, who is responsible for proper data stewardship? Entire teams, actually. Check the table below.
Role | Responsibility |
Data protection officer (DPO) | Oversees data protection strategies and ensures compliance with privacy laws and regulations. |
Data privacy engineer | Implements technical solutions (e.g., anonymization techniques, encryption methods) to protect user data. |
IT security teams | Implement robust security measures (e.g., firewalls, encryption methods) to prevent data breaches, unauthorized access, and other issues. |
Legal and compliance teams | Ensure that the company’s data practices comply with laws and regulations. |
Depending on the size and type of the business organization, the company might have a data governance committee. The committee is responsible for establishing and enforcing data governance policies, as well as implementing data management frameworks.
It’s more typical for larger organizations to have a committee since they handle significant amounts of sensitive data. Corporations, in particular, benefit from such centralized oversight and more risk management control.
Fairness and bias in AI models
We’ve seen that systematic and unfair discrimination in AI algorithms and models does exist. But where does it come from?
When the training data is not fully representative or contains historical prejudices, you cannot really expect it to be fair or accurate. This is why it’s important to diversify the training data.
For example, if you’re building an AI model for healthcare, you need to collect and incorporate medical records from different demographics, genders, socioeconomic backgrounds, and more. That’s the only way you can increase the model's accuracy and prevent misdiagnosis.
Let’s first take a look at different types of biases. Firstly, you need to be careful of selection and historical bias. Selection bias happens when the data used to train the model does not represent the entire population.
For example, an AI model for hiring might be trained only on resumes from a particular region or industry. This means it could unfairly disqualify good candidates just because they don’t fit the bill of the algorithm’s definition of success. It’s all because there are existing prejudices within the historical data.
Lastly, there’s measurement bias. Imagine there’s an AI model developed to predict patient outcomes. It’s been trained on data collected from various hospitals. However, the data from these hospitals are recorded differently– all because of the differences in measurement practices, technologies used, and staff training.
Because the data has been inconsistently recorded, the AI output might be inaccurate. Remember what we wrote earlier? AI is very dependent on the quality of data. And, inconsistent data can be dangerous.
Pre-processing, in-processing, and post-processing techniques are necessary to ensure fairness in AI models. You need to prepare, clean, and balance datasets before you feed them to AI. During model training, you need to integrate fairness constraints. And with post-processing techniques, you should remain objective and modify decision thresholds. You cannot train AI on data and just release it into the wild.
Importance of accountability in AI training
Precisely because we’re implementing AI in all kinds of industries, the stakes are getting higher. From finance to healthcare, we’re letting AI integrate deep into our communities. The upsides are very compelling, and so the risks and dangers fall into the background.
The importance of accountability in AI training cannot be overstated. You can find the roots of formalizing this accountability in early efforts by academic and research institutions, as well as international organizations.
For example, the European Commission released “Ethics Guidelines for Trustworthy AI” in 2019, and in 2016, the IEEE Global Initiative on Ethics of Autonomous and Intelligent Systems was launched.
Accountability is shared across the entire organization. From developers and regulatory bodies to data engineers and the leadership team–everyone plays their part. It’s a very complex system, and mistakes need to be minimized. When they do happen, it’s important to take ownership and do fast damage control. Why? Well, because accountability in AI training impacts public confidence in AI systems.
How to take data governance seriously
Data governance frameworks empower you to ethically manage data throughout the entire AI lifecycle–from data collection and pre-processing to model training and deployment. You need to think about managing and protecting data quality, data privacy, and data security.
As we already mentioned, the quality of your data directly impacts model accuracy and reliability, and it minimizes the chances of biases. Here’s a checklist for taking data governance seriously when developing AI models:
- Set clear data governance policies and make sure they align with legal regulations and ethical standards.
- Regularly review and adhere to data protection regulations (e.g., GDPR, CCPA).
- Monitor and maintain data quality through the entire lifecycle.
- Create clear guidelines on ethical use of data and promote the value of transparency.
- Protect data from unauthorized access, breaches, and misuse through security protocols.
Ethical AI development should not be taken lightly. And if it sounds like it’s a lot of work, that’s because it is a lot of work. But the good news is that you don’t have to do it alone.
Make the most of AI, ethically
In summary, to use AI ethically, you have to establish clear guidelines and respect regulations. Invest an effort to promote trust and explain to users how AI algorithms make decisions and extract insights. Keep everyone in the loop to prevent unpleasant surprises. It’s your responsibility to educate people on AI.
Undoubtedly, the benefits of AI are far bigger than the potential risks. However, human oversight is non-negotiable. If you’re ready to implement AI into your business but you’re not sure how to tackle complex regulations and model training, it makes sense to partner with an external tech expert. Want to learn more? Contact Vega IT for further information.