How to handle challenges when adopting AI in business
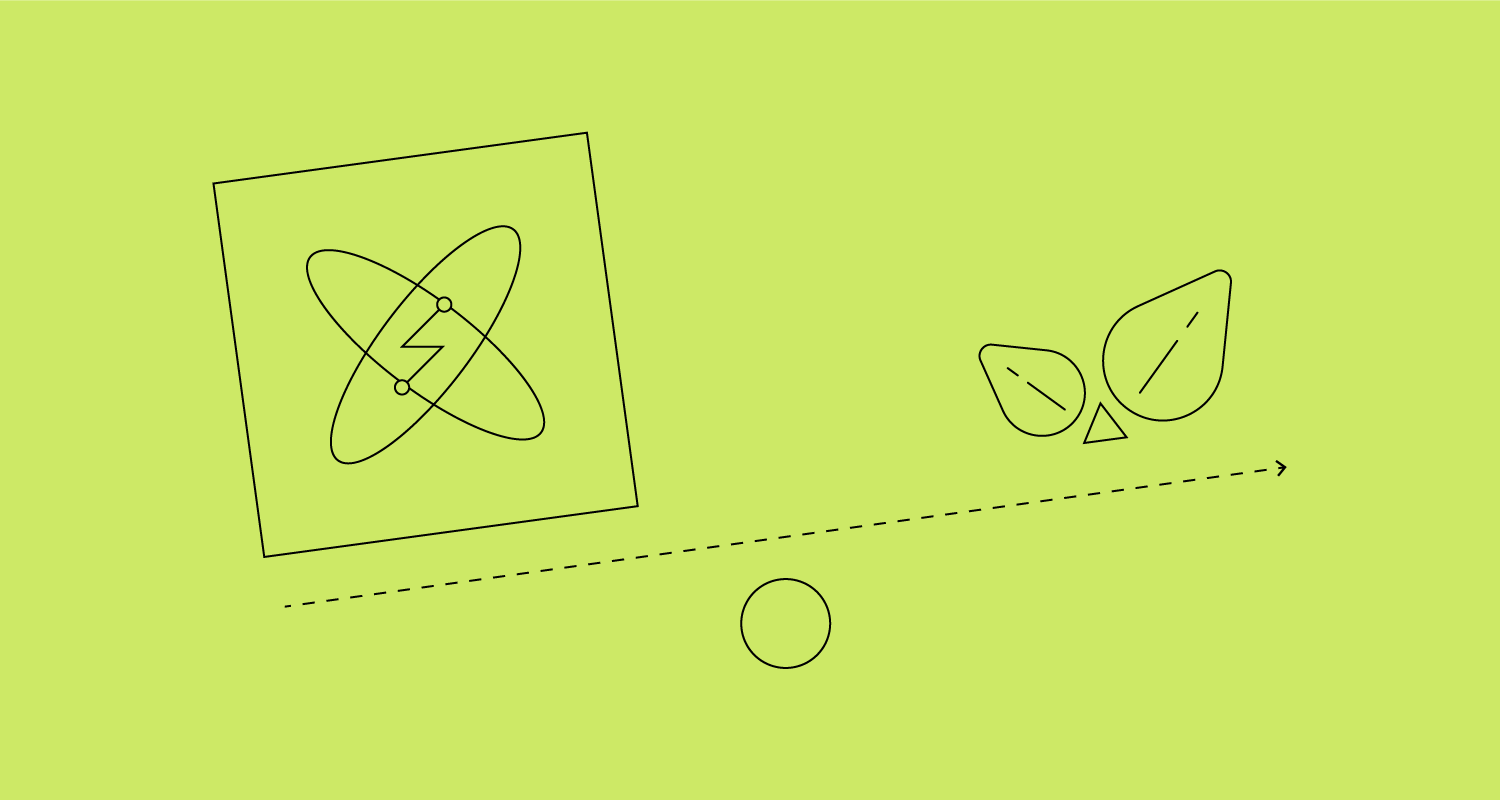
In 2014, Amazon was looking for an easier way to screen hundreds of candidates in their hiring process. So, they started working on AI-powered recruiting software. The system would rate candidates on a scale from 1 to 5, making it easier for HR personnel to spot suitable candidates. But that’s when things got controversial.
Just a couple of years after the official launch, Reuters published an article where they disclosed not only that Amazon had scrapped the project but also that the software was heavily biased against female candidates. That’s what you get when you feed the algorithm with historical data from the previous decade, and the majority of it compiles men’s CVs.
This is one of the many business mishaps that happened while companies tried to be more efficient by leveraging AI. If the model isn’t trained on data that reflects real-life situations, you get discrimination and inaccurate output.
So, how do you go about it? What can you do to address challenges when adopting AI properly?
The most common challenges in adopting AI in business
First things first, you have to be aware of the most common mistakes and challenges. Then, it gets a lot easier to address them properly and stay proactive. Here’s a list of the most common challenges:
- Data quality and reliability (as the Amazon example showed)
- Lack of proper pre-planning
- Issues with change management and lack of leadership support
- Resource allocation and ROI assessment
- Skill deficiency and lack of expertise
For each problem listed above, we have an antidote to recommend based on our decades' worth of experience.
Focus on data and think slowly
If there’s one thing you need to realize about adopting AI, it’s this: the amount of success and the extent to which you will reap the benefits of AI directly depends on the quality of your data.
The main way to de-risk your AI implementation in your business is to focus on data and to think slowly. Slow thinking is all about taking the time to look at the problem at hand from many angles, understand its complexity, and properly weigh the pros and cons of different paths you want to take.
In the context of AI adoption, it’s a common mistake to rush through the evaluation process that’s necessary to conclude the level of AI-readiness.
The first thing you need to evaluate is the data accuracy, reliability, and relevance. Here’s why:
- Accurate data empowers AI models to make precise predictions.
- Reliable data means that the data quality has been consistent over time, and this is important for the integrity of AI systems.
- Data relevancy is important because it directly impacts the effectiveness of your AI application (i.e., the inputs dictate the quality of insights AI synthesizes for you).
Additionally, data volume and variety influence the performance of machine learning models. In general, the more data you have, the better your chance of building a precise AI model.
While evaluating data, you should also audit it for bias. Data bias can lead to unfair and sometimes dangerous AI outcomes. Dr. Cathy O'Neil, author of “Weapons of Math Destruction,” spoke about how cautious we must be when choosing to trust big data.
In fact, AI models can be very opinionated – all because of the nature of the historical data they’ve been fed with. Such is the case with COMPAS, the controversial recidivism algorithm that unfairly predicted repeated crimes for minorities and Afro-Americans.
Identifying and addressing bias requires efforts from multiple disciplines. From data scientists and engineers to ethics and compliance officers to business leaders, diversity and inclusion specialists, and more, it truly takes a village.
Analyze existing systems to understand requirements
Another challenge associated with adopting AI in business is integrating it with your existing infrastructure. Integrating AI with legacy systems can be complex because of their rigid architecture and incompatibility with modern technologies.
To make the most of AI, you need to ensure that you have the technical capabilities to connect the old system with the model. There are three things to take into consideration when creating an integration roadmap – actions, timelines, and resources.
Here are the main steps you need to create an integration roadmap:
- Evaluate current infrastructure and capabilities.
- Define clear objectives.
- Map out the integration plan.
- Do a risk analysis.
- Define communication channels and monitor progress.
Mapping out the integration plan | |
Actions | Outline all tasks you need to finalize for a successful integration. This includes system upgrades, software development, data migration, and employee training. |
Timelines | Define realistic milestones and deadlines to ensure a healthy project pace. This is highly dependent on available resources. |
Resources | Think about the available budget, expertise, and technology you need. Sometimes, if you lack resources, you just need to adjust the timeline. It’s a trade-off. |
It’s very important to take a phased approach to implementation. When you divide the process into smaller, more manageable stages, you can effectively mitigate risks and work in iterations. This allows you to improve continuously and get the best possible results.
Move forward only when you get explicit leadership support
As if change management wasn’t hard enough, the initial support of the leadership team often fades out. If employees don’t have a person of authority to clearly state that AI is of strategic importance on a company level, it’s likely that you won’t see great adoption rates.
This is true even for cases where you tackle all technical challenges and there are clear benefits or using AI-driven workflows, for example.
So, what can you do? It’s important to get an executive sponsor on your team who will take responsibility for implementation, rollout, and impact measurement.
More often than not, employees might feel that the leadership team has little to no touch with what happens in the trenches. This is why it’s crucial to work on bridging the gap that gets in the way of successful AI adoption. The best way to do it? Involve employees in the process.
It is absurd to work on solutions that your employees are expected to use without any type of input from their side. Work on creating a cross-team pod that will help everyone involved understand the company-wide benefits. There are two principles to remember here:
- Communicate early and often to make sure people understand what’s going on and why the company’s doing it.
- Prioritize explaining the “what’s in it for me” to manage change within your organization successfully.
Don’t compromise on detailed cost-benefit analysis
Artificial intelligence exists in so many shapes and sizes. This means that the scope of implementation can also vary depending on your needs and investments.
It’s important not to rush things, or you might find yourself in a situation where you have already wasted too much money, and the return on investment does not look good. Smaller businesses with scrappy teams are typically the main victims of this.
In a nutshell, a cost-benefit analysis compares the costs of AI implementation with anticipated benefits (e.g., saved time, increased productivity, a boost in sales). The goal is to understand whether the investment can be justified and to predict the impact.
In the table below, you can see an example of what you’ll be taking into consideration:
Initial costs | Operational costs | Potential benefits |
|
|
|
A good rule of thumb is to prioritize investments by thinking of what needs scale as soon as possible. This means that you identify high-impact areas, start small, and then use pilot projects to justify larger investments. You need to get strategic alignment on what’s an acceptable ROI and how you’ll measure it, and it’s not wise to go all in right away.
Get the right talent to drive AI
Many companies are severely undereducated about the true power of AI. Some might even put an equal sign between AI as a field and generative AI tools such as ChatGPT. Such shortsightedness is costing companies thousands of dollars, contributing to the following fallacy: we don’t need AI experts on our team.
If you don’t want to hire internally, that’s fine (at least for now). However, you need proper employee training and support, regardless of their immediate area of expertise. Without users that understand what AI can do for them, truly harnessing the power of technology is impossible.
There are numerous options for employee training. There are AI consultants who organize workshops and seminars within an organization to ensure people understand the value of AI and how to use it.
You can also outsource AI competence building. It’s your responsibility to provide resources to employees and spark their curiosity so that they continue learning on their own as well. It’s a never-ending process. The AI community is large, and it continues to grow.
How to avoid the biggest mistake?
When adopting AI in your business, the biggest mistake you can make is turning a blind eye to these challenges. You need to ensure your data is accurate and reliable because that dictates the output AI will give you. Likewise, you have to reach internal alignment on strategy, budget, change management, and the culture you’ll build around innovation.
Do not think you’re immune to these pitfalls. A lot of moving parts need to fall into place for AI to make an impact on your business. Leaders are anxious about it, and rightfully so. It takes a lot of investment, effort, and pre-planning to see the benefits of AI. But you don’t have to do it alone.
If you need help determining the best path forward, rely on experts with a proven track record in AI implementation. Form partnerships to compensate for skill deficiencies so that you can focus on your area of expertise and shorten the time-to-value of implementing AI.